Data Preparation
Contents
Data Preparation#
author: Hamid Ali Syed
email: hamidsyed37[at]gmail[dot]com
import warnings
warnings.filterwarnings("ignore")
import requests
from bs4 import BeautifulSoup
import pandas as pd
import numpy as np
Data collection#
Let’s do some Web Scrapping#
Let’s do some Web Scrapping. We can scrap the radar site information from the website of the Indian Meteorological Department (IMD) and extract location information from the HTML using BeautifulSoup. Then, we can clean and transform the extracted data into a Pandas DataFrame, with longitude and latitude coordinates for each location. Finally, we can plot the DataFrame as a scatter plot using longitude and latitude as x and y axes, respectively.
url = "https://mausam.imd.gov.in/imd_latest/contents/index_radar.php"
response = requests.get(url)
soup = BeautifulSoup(response.text, "html.parser")
# extract the relevant part of the HTML
images_html = soup.find_all("script")[-2].text.split("images: [")[0].split("],\n")[0]
# split the HTML into individual locations and extract the relevant information
locations = []
for image in soup.find_all("script")[-2].text.split("images: [")[0].split("],\n")[0].split("{")[1:]:
location_dict = {}
for line in image.split("\n"):
if "title" in line:
location_dict["title"] = line.split(": ")[-1].strip(',')
elif "latitude" in line:
location_dict["latitude"] = line.split(": ")[-1].strip(',')
elif "longitude" in line:
location_dict["longitude"] = line.split(": ")[-1].strip(',')
locations.append(location_dict)
# create a DataFrame from the list of dictionaries
df = pd.DataFrame(locations)
df = df.dropna()
df['title'] = df['title'].str.strip(", ").str.strip('"')
df['longitude'] = df['longitude'].str.strip(", ").str.strip('longitude":')
df['latitude'] = df['latitude'].astype(float)
df['longitude'] = df['longitude'].astype(float)
df['title'].replace("Goa", "Panaji", inplace=True)
df.plot(kind='scatter', x='longitude', y='latitude')
df
title | latitude | longitude | |
---|---|---|---|
2 | Bhopal | 23.259900 | 77.412600 |
3 | Agartala | 23.831500 | 91.286800 |
4 | Delhi | 28.563200 | 77.191200 |
5 | Bhuj | 23.242000 | 69.666900 |
6 | Chennai | 13.082700 | 80.270700 |
7 | Panaji | 15.490900 | 73.827800 |
8 | Gopalpur | 19.264700 | 84.862000 |
9 | Hyderabad | 17.385000 | 78.486700 |
10 | Jaipur | 26.912400 | 75.787300 |
11 | Kolkata | 22.572600 | 88.363900 |
12 | Kochi | 9.931200 | 76.267300 |
13 | Karaikal | 10.925400 | 79.838000 |
14 | Lucknow | 26.846700 | 80.946200 |
15 | Machilipatnam | 16.190500 | 81.136200 |
16 | Mumbai | 19.076000 | 72.877700 |
17 | Nagpur | 21.145800 | 79.088200 |
18 | Sohra | 25.270200 | 91.732300 |
19 | Patiala | 30.339800 | 76.386900 |
20 | Patna | 25.594100 | 85.137600 |
21 | Srinagar | 34.083656 | 74.797371 |
22 | Jammu | 32.926600 | 74.857000 |
23 | Thiruvananthapuram | 8.524100 | 76.936600 |
24 | Visakhapatnam | 17.686800 | 83.218500 |
25 | Mohanbari | 27.472800 | 94.912000 |
26 | Paradip | 20.316600 | 86.611400 |
27 | Sriharikota | 13.725900 | 80.226600 |
28 | Jot | 32.486800 | 76.059300 |
29 | Murari | 30.789800 | 78.917850 |
30 | Palam | 28.590100 | 77.088800 |
31 | Mukteshwar | 29.460400 | 79.655800 |
32 | Veravali | 19.734300 | 72.876300 |
33 | Kufri | 31.097800 | 77.267800 |
34 | Surkandaji | 30.411400 | 78.288500 |
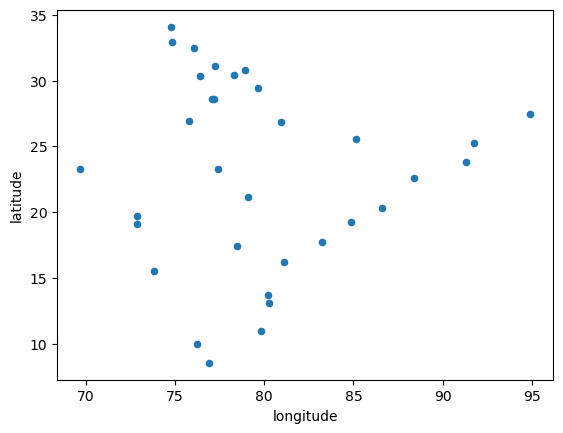
We have procured the name, lat, & lon info of all the radar sites and is saved in df
. Now, let’s search for their frequency bands. I have found a webpage on the IMD website that contains this information for most of the radars. Let’s make a request to a URL and create a BeautifulSoup object to parse the HTML content. It will find a table on the page, then we can extract the headers and rows of the table, and create a Pandas DataFrame df2
from the table data.
Drop the "S No" column, clean up the "Type of DWR" and "DWR Station" columns by removing certain text, and replace some values in the "DWR Station" column.
# make a request to the URL
url = "https://mausam.imd.gov.in/imd_latest/contents/imd-dwr-network.php"
response = requests.get(url)
# create a BeautifulSoup object
soup = BeautifulSoup(response.content, "html.parser")
# find the table on the page
table = soup.find("table")
# extract the table headers
headers = [header.text.strip() for header in table.find_all("th")]
# extract the table rows
rows = []
for row in table.find_all("tr")[1:]:
cells = [cell.text.strip() for cell in row.find_all("td")]
rows.append(cells)
# create a DataFrame from the table data
df2 = pd.DataFrame(rows, columns=headers)
df2.drop("S No", axis=1, inplace=True)
df2['Type of DWR'] = df2['Type of DWR'].str.replace(' - Band', '')
df2['DWR Station'].replace('Delhi (Palam)', 'Palam', inplace=True)
df2['DWR Station'] = df2['DWR Station'].str.replace('\(ISRO\)', '').str.replace('\(Mausam Bhawan\)',
'').str.strip()
df2
DWR Station | State | Type of DWR | |
---|---|---|---|
0 | Agartala | Tripura | S |
1 | Bhopal | Madhya Pradesh | S |
2 | Bhuj | Gujarat | S |
3 | Chennai | Tamil Nadu | S |
4 | Cherrapunjee | Meghalaya | S |
5 | Palam | Delhi | S |
6 | Panaji | Goa | S |
7 | Gopalpur | Odisha | S |
8 | Hyderabad | Telangana | S |
9 | Jaipur | Rajasthan | C |
10 | Kolkata | West Bengal | S |
11 | Kochi | Kerala | S |
12 | Karaikal | Tamil Nadu | S |
13 | Lucknow | Uttar Pradesh | S |
14 | Machilipatnam | Andhra Pradesh | S |
15 | Mohanbari | Assam | S |
16 | Mumbai | Maharashtra | S |
17 | Nagpur | Maharashtra | S |
18 | New Delhi | Delhi | C |
19 | Paradip | Odisha | S |
20 | Patiala | Punjab | S |
21 | Patna | Bihar | S |
22 | Srinagar | Jammu and Kashmir | X |
23 | Thiruvananthapuram | Kerala | C |
24 | Visakhapatnam | Andhra Pradesh | S |
Let’s merge two previously created Pandas DataFrames, df
and df2
, using the “title” and “DWR Station” columns as keys, respectively. It will drop the “DWR Station” column, rename the “Type of DWR” column as “Band”, and replace some values in the “title” column. The code will count the number of NaN values in the “Band” column, print this count, and return the resulting merged DataFrame.
merged_df = df.merge(df2, left_on='title', right_on='DWR Station', how='left')
merged_df = merged_df.drop(columns=['DWR Station'])
merged_df = merged_df.rename(columns={'Type of DWR': 'Band'})
merged_df['title'].replace("Goa", "Panaji", inplace=True)
num_nans = merged_df['Band'].isna().sum()
print(num_nans)
merged_df
10
title | latitude | longitude | State | Band | |
---|---|---|---|---|---|
0 | Bhopal | 23.259900 | 77.412600 | Madhya Pradesh | S |
1 | Agartala | 23.831500 | 91.286800 | Tripura | S |
2 | Delhi | 28.563200 | 77.191200 | NaN | NaN |
3 | Bhuj | 23.242000 | 69.666900 | Gujarat | S |
4 | Chennai | 13.082700 | 80.270700 | Tamil Nadu | S |
5 | Panaji | 15.490900 | 73.827800 | Goa | S |
6 | Gopalpur | 19.264700 | 84.862000 | Odisha | S |
7 | Hyderabad | 17.385000 | 78.486700 | Telangana | S |
8 | Jaipur | 26.912400 | 75.787300 | Rajasthan | C |
9 | Kolkata | 22.572600 | 88.363900 | West Bengal | S |
10 | Kochi | 9.931200 | 76.267300 | Kerala | S |
11 | Karaikal | 10.925400 | 79.838000 | Tamil Nadu | S |
12 | Lucknow | 26.846700 | 80.946200 | Uttar Pradesh | S |
13 | Machilipatnam | 16.190500 | 81.136200 | Andhra Pradesh | S |
14 | Mumbai | 19.076000 | 72.877700 | Maharashtra | S |
15 | Nagpur | 21.145800 | 79.088200 | Maharashtra | S |
16 | Sohra | 25.270200 | 91.732300 | NaN | NaN |
17 | Patiala | 30.339800 | 76.386900 | Punjab | S |
18 | Patna | 25.594100 | 85.137600 | Bihar | S |
19 | Srinagar | 34.083656 | 74.797371 | Jammu and Kashmir | X |
20 | Jammu | 32.926600 | 74.857000 | NaN | NaN |
21 | Thiruvananthapuram | 8.524100 | 76.936600 | Kerala | C |
22 | Visakhapatnam | 17.686800 | 83.218500 | Andhra Pradesh | S |
23 | Mohanbari | 27.472800 | 94.912000 | Assam | S |
24 | Paradip | 20.316600 | 86.611400 | Odisha | S |
25 | Sriharikota | 13.725900 | 80.226600 | NaN | NaN |
26 | Jot | 32.486800 | 76.059300 | NaN | NaN |
27 | Murari | 30.789800 | 78.917850 | NaN | NaN |
28 | Palam | 28.590100 | 77.088800 | Delhi | S |
29 | Mukteshwar | 29.460400 | 79.655800 | NaN | NaN |
30 | Veravali | 19.734300 | 72.876300 | NaN | NaN |
31 | Kufri | 31.097800 | 77.267800 | NaN | NaN |
32 | Surkandaji | 30.411400 | 78.288500 | NaN | NaN |
Since there are NaN values in the “State” column, we can find the state names using lat and lon info. We can use the Cartopy library to create a map of India with state boundaries and labels. Then we will create a pandas DataFrame gdf
containing the latitude and longitude coordinates of each state and union territory, and try to map the names of these places in the merged_df
import cartopy.io.shapereader as shpreader
import geopandas as gpd
# Load the Natural Earth dataset
states_shp = shpreader.natural_earth(resolution='10m',
category='cultural',
name='admin_1_states_provinces')
import cartopy.crs as ccrs
import cartopy.io.shapereader as shpreader
import cartopy.feature as feat
import matplotlib.pyplot as plt
import matplotlib.patheffects as PathEffects
# get the data
fn = shpreader.natural_earth(
resolution='10m', category='cultural',
name='admin_1_states_provinces',
)
reader = shpreader.Reader(fn)
states = [x for x in reader.records() if x.attributes["admin"] == "India"]
states_geom = feat.ShapelyFeature([x.geometry for x in states], ccrs.PlateCarree())
data_proj = ccrs.PlateCarree()
# create the plot
fig, ax = plt.subplots(
figsize=(10,10), dpi=70, facecolor="w",
subplot_kw=dict(projection=data_proj),
)
ax.add_feature(feat.BORDERS, color="k", lw=0.1)
# ax.add_feature(feat.COASTLINE, color="k", lw=0.2)
ax.set_extent([60, 100, 5, 35], crs=ccrs.Geodetic())
ax.add_feature(states_geom, facecolor="none", edgecolor="k")
# # add the names
for state in states:
lon = state.geometry.centroid.x
lat = state.geometry.centroid.y
name = state.attributes["name"]
ax.text(
lon, lat, name, size=7, transform=data_proj, ha="center", va="center",
path_effects=[PathEffects.withStroke(linewidth=5, foreground="w")]
)
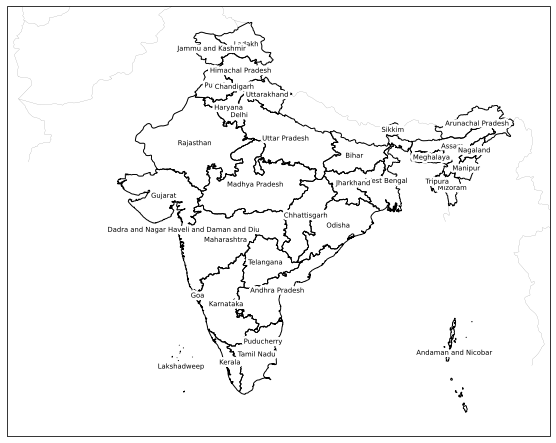
locs = {}
for state in states:
lon = state.geometry.centroid.x
lat = state.geometry.centroid.y
name = state.attributes["name"]
locs[name] = {"lat": lat, "lon": lon}
gdf = pd.DataFrame(locs, ).T
gdf.reset_index(inplace=True)
gdf = gdf.rename({'index':'state'}, axis=1)
gdf.index = np.arange(1, len(gdf) + 1)
gdf
state | lat | lon | |
---|---|---|---|
1 | Ladakh | 33.885957 | 77.634965 |
2 | Arunachal Pradesh | 28.035900 | 94.660514 |
3 | Sikkim | 27.572023 | 88.448173 |
4 | West Bengal | 23.805249 | 87.972564 |
5 | Assam | 26.356938 | 92.831287 |
6 | Uttarakhand | 30.163721 | 79.196121 |
7 | Nagaland | 26.059820 | 94.448403 |
8 | Manipur | 24.730388 | 93.861591 |
9 | Mizoram | 23.292031 | 92.819139 |
10 | Tripura | 23.754753 | 91.728537 |
11 | Meghalaya | 25.536122 | 91.287036 |
12 | Punjab | 30.848293 | 75.404354 |
13 | Rajasthan | 26.592714 | 73.834251 |
14 | Gujarat | 22.712829 | 71.558165 |
15 | Himachal Pradesh | 31.936310 | 77.220849 |
16 | Jammu and Kashmir | 33.557813 | 75.079701 |
17 | Bihar | 25.662102 | 85.604230 |
18 | Uttar Pradesh | 26.934734 | 80.541922 |
19 | Andhra Pradesh | 15.721672 | 79.924211 |
20 | Odisha | 20.517113 | 84.414536 |
21 | Dadra and Nagar Haveli and Daman and Diu | 20.199380 | 72.992494 |
22 | Maharashtra | 19.460457 | 76.111348 |
23 | Goa | 15.352195 | 74.045828 |
24 | Karnataka | 14.719826 | 76.155168 |
25 | Kerala | 10.423815 | 76.424882 |
26 | Puducherry | 11.960162 | 78.885684 |
27 | Tamil Nadu | 11.014705 | 78.402304 |
28 | Lakshadweep | 10.120942 | 72.827601 |
29 | Andaman and Nicobar | 11.133776 | 92.975529 |
30 | Jharkhand | 23.642010 | 85.533387 |
31 | Delhi | 28.660865 | 77.107946 |
32 | Chandigarh | 30.743535 | 76.768380 |
33 | Madhya Pradesh | 23.539092 | 78.292780 |
34 | Chhattisgarh | 21.255901 | 82.033368 |
35 | Haryana | 29.208370 | 76.336467 |
36 | Telangana | 17.796650 | 79.050764 |
# merged_df.sort_values(by=['latitude', 'longitude'], ascending=False)
merged_df = df.merge(df2, left_on='title', right_on='DWR Station', how='left')
merged_df = merged_df.drop(columns=['DWR Station'])
merged_df = merged_df.rename(columns={'Type of DWR': 'Band'})
merged_df
title | latitude | longitude | State | Band | |
---|---|---|---|---|---|
0 | Bhopal | 23.259900 | 77.412600 | Madhya Pradesh | S |
1 | Agartala | 23.831500 | 91.286800 | Tripura | S |
2 | Delhi | 28.563200 | 77.191200 | NaN | NaN |
3 | Bhuj | 23.242000 | 69.666900 | Gujarat | S |
4 | Chennai | 13.082700 | 80.270700 | Tamil Nadu | S |
5 | Panaji | 15.490900 | 73.827800 | Goa | S |
6 | Gopalpur | 19.264700 | 84.862000 | Odisha | S |
7 | Hyderabad | 17.385000 | 78.486700 | Telangana | S |
8 | Jaipur | 26.912400 | 75.787300 | Rajasthan | C |
9 | Kolkata | 22.572600 | 88.363900 | West Bengal | S |
10 | Kochi | 9.931200 | 76.267300 | Kerala | S |
11 | Karaikal | 10.925400 | 79.838000 | Tamil Nadu | S |
12 | Lucknow | 26.846700 | 80.946200 | Uttar Pradesh | S |
13 | Machilipatnam | 16.190500 | 81.136200 | Andhra Pradesh | S |
14 | Mumbai | 19.076000 | 72.877700 | Maharashtra | S |
15 | Nagpur | 21.145800 | 79.088200 | Maharashtra | S |
16 | Sohra | 25.270200 | 91.732300 | NaN | NaN |
17 | Patiala | 30.339800 | 76.386900 | Punjab | S |
18 | Patna | 25.594100 | 85.137600 | Bihar | S |
19 | Srinagar | 34.083656 | 74.797371 | Jammu and Kashmir | X |
20 | Jammu | 32.926600 | 74.857000 | NaN | NaN |
21 | Thiruvananthapuram | 8.524100 | 76.936600 | Kerala | C |
22 | Visakhapatnam | 17.686800 | 83.218500 | Andhra Pradesh | S |
23 | Mohanbari | 27.472800 | 94.912000 | Assam | S |
24 | Paradip | 20.316600 | 86.611400 | Odisha | S |
25 | Sriharikota | 13.725900 | 80.226600 | NaN | NaN |
26 | Jot | 32.486800 | 76.059300 | NaN | NaN |
27 | Murari | 30.789800 | 78.917850 | NaN | NaN |
28 | Palam | 28.590100 | 77.088800 | Delhi | S |
29 | Mukteshwar | 29.460400 | 79.655800 | NaN | NaN |
30 | Veravali | 19.734300 | 72.876300 | NaN | NaN |
31 | Kufri | 31.097800 | 77.267800 | NaN | NaN |
32 | Surkandaji | 30.411400 | 78.288500 | NaN | NaN |
from math import radians, sin, cos, sqrt, asin
# Function to calculate the haversine distance between two coordinates in km
def haversine(lat1, lon1, lat2, lon2):
R = 6371 # radius of Earth in km
dLat = radians(lat2 - lat1)
dLon = radians(lon2 - lon1)
lat1 = radians(lat1)
lat2 = radians(lat2)
a = sin(dLat/2)**2 + cos(lat1)*cos(lat2)*sin(dLon/2)**2
c = 2*asin(sqrt(a))
return R*c
# Loop through each row in merged_df
for i, row in merged_df.iterrows():
if pd.isna(row["State"]):
min_dist = float('inf')
closest_state = ""
# Loop through each row in gdf to find the closest one
for j, gdf_row in gdf.iterrows():
dist = haversine(row["latitude"], row["longitude"], gdf_row["lat"], gdf_row["lon"])
if dist < min_dist:
min_dist = dist
closest_state = gdf_row["state"]
merged_df.at[i, "State"] = closest_state
merged_df.sort_values(by = "latitude", ascending=False)
title | latitude | longitude | State | Band | |
---|---|---|---|---|---|
19 | Srinagar | 34.083656 | 74.797371 | Jammu and Kashmir | X |
20 | Jammu | 32.926600 | 74.857000 | Jammu and Kashmir | NaN |
26 | Jot | 32.486800 | 76.059300 | Himachal Pradesh | NaN |
31 | Kufri | 31.097800 | 77.267800 | Chandigarh | NaN |
27 | Murari | 30.789800 | 78.917850 | Uttarakhand | NaN |
32 | Surkandaji | 30.411400 | 78.288500 | Uttarakhand | NaN |
17 | Patiala | 30.339800 | 76.386900 | Punjab | S |
29 | Mukteshwar | 29.460400 | 79.655800 | Uttarakhand | NaN |
28 | Palam | 28.590100 | 77.088800 | Delhi | S |
2 | Delhi | 28.563200 | 77.191200 | Delhi | NaN |
23 | Mohanbari | 27.472800 | 94.912000 | Assam | S |
8 | Jaipur | 26.912400 | 75.787300 | Rajasthan | C |
12 | Lucknow | 26.846700 | 80.946200 | Uttar Pradesh | S |
18 | Patna | 25.594100 | 85.137600 | Bihar | S |
16 | Sohra | 25.270200 | 91.732300 | Meghalaya | NaN |
1 | Agartala | 23.831500 | 91.286800 | Tripura | S |
0 | Bhopal | 23.259900 | 77.412600 | Madhya Pradesh | S |
3 | Bhuj | 23.242000 | 69.666900 | Gujarat | S |
9 | Kolkata | 22.572600 | 88.363900 | West Bengal | S |
15 | Nagpur | 21.145800 | 79.088200 | Maharashtra | S |
24 | Paradip | 20.316600 | 86.611400 | Odisha | S |
30 | Veravali | 19.734300 | 72.876300 | Dadra and Nagar Haveli and Daman and Diu | NaN |
6 | Gopalpur | 19.264700 | 84.862000 | Odisha | S |
14 | Mumbai | 19.076000 | 72.877700 | Maharashtra | S |
22 | Visakhapatnam | 17.686800 | 83.218500 | Andhra Pradesh | S |
7 | Hyderabad | 17.385000 | 78.486700 | Telangana | S |
13 | Machilipatnam | 16.190500 | 81.136200 | Andhra Pradesh | S |
5 | Panaji | 15.490900 | 73.827800 | Goa | S |
25 | Sriharikota | 13.725900 | 80.226600 | Andhra Pradesh | NaN |
4 | Chennai | 13.082700 | 80.270700 | Tamil Nadu | S |
11 | Karaikal | 10.925400 | 79.838000 | Tamil Nadu | S |
10 | Kochi | 9.931200 | 76.267300 | Kerala | S |
21 | Thiruvananthapuram | 8.524100 | 76.936600 | Kerala | C |
# Merge merged_df and df2 on the "State" column
merged_df_with_band = pd.merge(merged_df, df2[['State', 'Type of DWR']], on='State', how='left')
# Replace NaN values in the "Band" column with corresponding values from the "Type of DWR" column
merged_df_with_band['Band'].fillna(merged_df_with_band['Type of DWR'], inplace=True)
# Drop the "Type of DWR" column
merged_df_with_band.drop('Type of DWR', axis=1, inplace=True)
merged_df_with_band.drop(2, inplace=True)
merged_df_with_band.drop_duplicates("latitude", inplace=True)
merged_df_with_band.drop_duplicates("longitude", inplace=True)
merged_df_with_band.sort_values(by="latitude", ascending=False, inplace=True)
merged_df_with_band.index = np.arange(1, len(merged_df_with_band)+1, 1)
f_df = merged_df_with_band.copy()
f_df.loc[f_df['title'] == 'Veravali', 'Band'] = 'C'
nan_mask = f_df['Band'].isna()
nan_df = f_df[nan_mask]
nan_df.loc[:, 'Band'] = nan_df['Band'].fillna('X')
f_df.update(nan_df)
f_df.rename(columns={'title': 'Site',
"latitude": "Latitude",
"longitude":"Longitude"}, inplace=True)
f_df.attrs["Range"]={"C":250,
"X":100,
"S":250
}
f_df.to_csv("IMD_Radar_Sites_2022.csv")